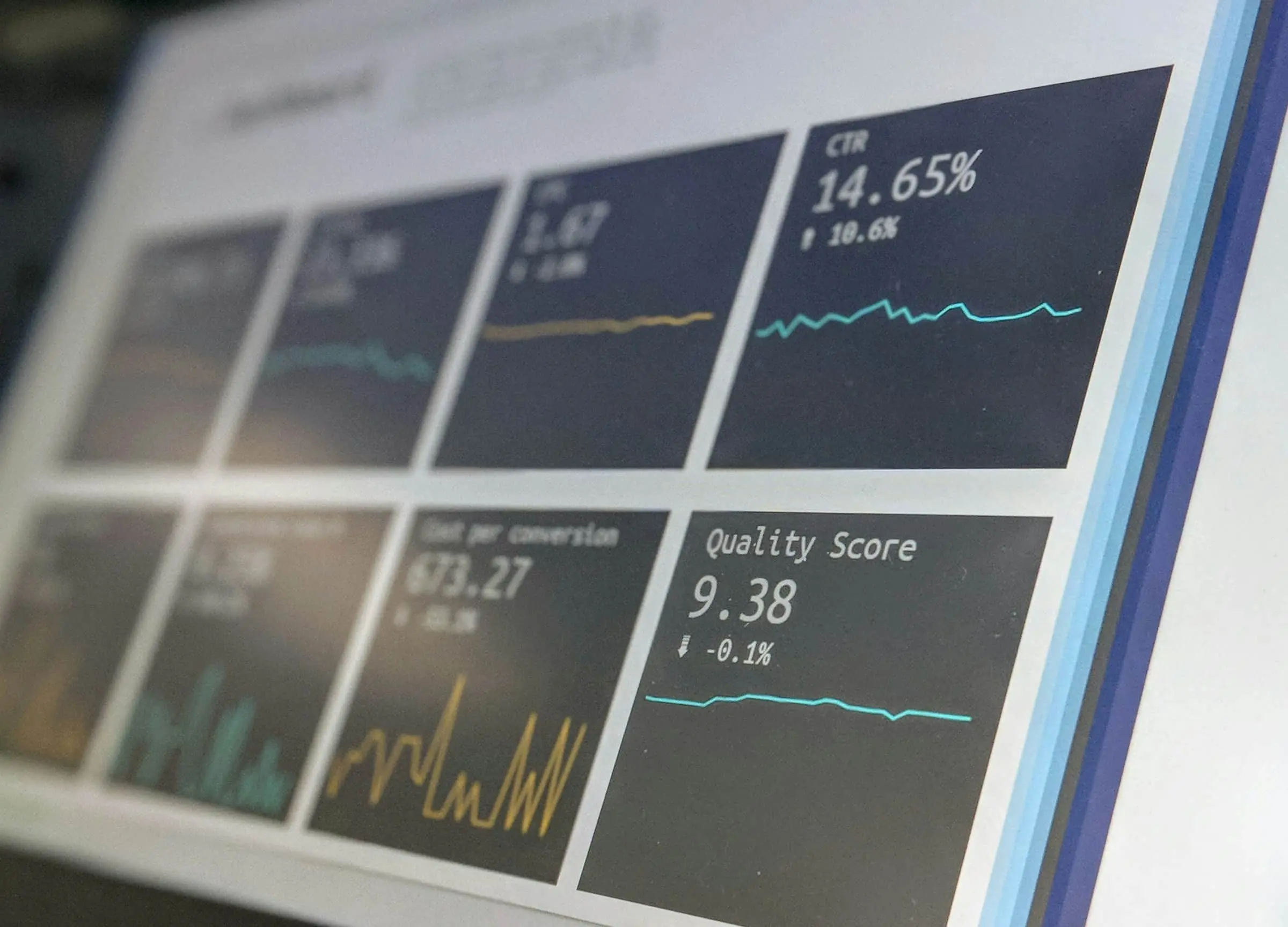
AI and Data-Driven Marketing: How to Make Smarter Decisions
The modern marketer must harness the power of artificial intelligence (AI) and data analytics to make informed decisions that deliver measurable results. This shift toward data-driven marketing isn’t just a trend—it’s a fundamental transformation in how businesses connect with their customers and optimize their marketing efforts.
The Evolution of Marketing Decision-Making
Traditional marketing relied heavily on creative intuition, experience, and broad demographic targeting. While these elements remain valuable, they now serve as just one part of a more sophisticated approach. The evolution to data-driven marketing has been dramatic:
From: “I think our customers would like this campaign.”
To: “Our data indicates this campaign will increase engagement by 27% among our high-value customer segments.”
This transformation has been accelerated by advances in AI technology, making powerful analytics accessible to organizations of all sizes. Let’s explore how AI and data are revolutionizing marketing decision-making and how you can implement these approaches in your organization.
What Makes Marketing Truly Data-Driven?
Data-driven marketing goes beyond simply collecting information. It involves:
- Systematic data collection across customer touchpoints
- Advanced analysis using AI and machine learning to identify patterns and insights
- Actionable intelligence that directly informs marketing strategy and tactics
- Continuous measurement of outcomes to refine future decisions
The key difference between traditional data usage and true data-driven marketing lies in how comprehensively data informs the entire marketing process—from initial audience research to campaign optimization and performance evaluation.
Key Areas Where AI Enhances Marketing Decisions
Customer Segmentation and Targeting
AI excels at identifying patterns in customer behavior that would be impossible for humans to detect manually. Rather than relying on basic demographic segments, AI can create dynamic customer clusters based on:
- Purchase history and browsing behavior
- Content preferences and engagement patterns
- Predicted lifetime value and churn risk
- Cross-channel interaction data
Real-world application: An e-commerce retailer implemented AI-powered segmentation that identified a previously unrecognized high-value customer group who primarily shopped during late-night hours. Creating targeted campaigns for this segment increased their revenue by 34%.
Content and Creative Optimization
Creating effective marketing content has traditionally been considered primarily an art form. AI transforms this process by providing data-driven insights for:
- Topic selection based on search intent and audience interest
- Headline optimization for maximum engagement
- Image and design element performance prediction
- Content personalization at scale
Real-world application: A B2B software company used AI to analyze their highest-performing blog content and identified specific phrases, formats, and topics that consistently generated leads. Applying these insights to new content increased their conversion rate by 41%.
Channel and Budget Allocation
Determining where to invest marketing resources is one of the most critical decisions marketers face. AI helps optimize these decisions by:
- Predicting channel performance for specific campaign goals
- Dynamically reallocating budget based on real-time results
- Identifying optimal timing for different marketing activities
- Revealing synergies between channels and touchpoints
Real-world application: A retail brand implemented AI-driven media mix modeling that revealed diminishing returns in their paid social spending but untapped potential in email marketing. Reallocating 20% of their budget based on these insights improved their overall ROI by 28%.
Conversion Path Optimization
Understanding how customers move from awareness to purchase is crucial for effective marketing. AI helps marketers optimize this journey by:
- Identifying friction points in the conversion process
- Recommending personalized next-best-actions for each customer
- Predicting which leads are most likely to convert
- Determining optimal frequency and timing of touchpoints
Real-world application: A travel company used AI to analyze customer booking journeys and discovered that prospects who received a specific sequence of emails and retargeting ads converted at 3x the rate of others. Implementing this sequence as an automated workflow increased bookings by 52%.
How to Implement AI-Driven Marketing Decision-Making
Transforming your marketing to become more data-driven and AI-enabled doesn’t happen overnight. Here’s a pragmatic roadmap:
1. Audit Your Current Data Capabilities
Before implementing new AI tools, assess your current data ecosystem:
- What customer data are you already collecting?
- How accessible and clean is this data?
- What are your most significant data gaps?
- How is data currently being used in decision-making?
This audit will help you identify your starting point and prioritize improvements.
2. Define Key Business Questions
AI is most valuable when applied to specific, high-impact business questions. Examples include:
- Which customer segments offer the highest growth potential?
- How can we reduce customer acquisition costs while maintaining quality?
- What content resonates most with our high-value prospects?
- Which touchpoints have the greatest influence on purchase decisions?
Clearly defining these questions ensures your data initiatives remain focused on business outcomes.
3. Start with Quick Wins
Look for opportunities to implement AI in areas where:
- You already have sufficient quality data
- The potential business impact is significant
- Implementation complexity is manageable
- Results can be quickly measured
These early successes will build organizational confidence and momentum.
4. Build a Data-Friendly Culture
Technical tools alone won’t transform your marketing. You also need to:
- Encourage data literacy across marketing teams
- Establish processes for incorporating data insights into decisions
- Create feedback loops between AI predictions and actual outcomes
- Balance data-driven insights with creative expertise
Organizations that successfully integrate AI into marketing decisions recognize that humans and machines have complementary strengths.
5. Scale Gradually
As your organization becomes more comfortable with data-driven decision-making, gradually expand your AI applications:
- Implement more sophisticated predictive models
- Connect data across more touchpoints and systems
- Automate routine decision processes
- Explore advanced applications like natural language generation and image recognition
Common Challenges and How to Overcome Them
Data Quality and Integration Issues
Challenge: Fragmented data across multiple systems with inconsistent formats and quality issues.
Solution: Invest in customer data platforms (CDPs) or data integration tools that create unified customer profiles. Implement data governance practices to ensure ongoing data quality.
Balancing Automation and Human Judgment
Challenge: Over-reliance on AI recommendations without considering contextual factors or creative intuition.
Solution: Use AI as a decision support tool rather than replacing human judgment entirely. Create processes where AI insights inform human decisions rather than dictate them.
Privacy and Ethical Considerations
Challenge: Navigating increasingly complex privacy regulations while still leveraging customer data effectively.
Solution: Implement privacy-by-design principles, be transparent with customers about data usage, and explore techniques like federated learning that provide insights without compromising individual privacy.
Skills and Knowledge Gaps
Challenge: Marketing teams lacking technical expertise to implement and interpret AI tools.
Solution: Provide training on data literacy, hire analytics translators who can bridge technical and marketing domains, and consider partnerships with specialized AI marketing agencies.
The Future of AI in Marketing Decision-Making
As AI continues to evolve, we can expect several important developments:
Predictive to Prescriptive Analytics
Marketing AI will move beyond predicting outcomes to actively recommending specific actions to achieve desired results. For example, rather than just forecasting campaign performance, AI will suggest specific creative changes and targeting adjustments to improve outcomes.
Seamless Cross-Channel Orchestration
Advanced AI systems will coordinate messaging across channels in real-time, ensuring consistent experiences while optimizing each touchpoint based on individual customer preferences and behaviors.
Augmented Creativity
Rather than replacing creative processes, AI will enhance them by generating variations, testing performance, and identifying elements that resonate with specific audiences. This human-AI collaboration will produce more effective creative at scale.
Ethical AI and Trusted Marketing
As consumer privacy concerns grow, successful organizations will implement transparent AI systems that build trust while still delivering personalization. Explainable AI that can articulate the reasoning behind recommendations will become increasingly important.